The world of technology is constantly changing, and two of the most dynamic areas are Artificial Intelligence (AI) and Industry 4.0 (I4.0). Both have the potential to fundamentally change the way we work and live. But as enthusiasm for these technologies grows, it's important to take a critical look at the current hype and real-world impact.

The hype about AI: Justified or exaggerated?
AI has attracted a lot of attention in recent years. From self-driving cars to intelligent assistants, AI applications seem limitless. But as with any new technology, there is a discrepancy between expectation and reality. The Gartner Hype Cycle shows that AI technologies such as generative AI and decision intelligence are currently at the peak of inflated expectations. This means that many AI applications are still in the development phase, and their true scope and reliability have yet to be proven.
Is AI a game changer?
Yes, AI has the potential to be a game changer. It can automate processes, improve decision-making and enable new business models. However, it is important to remain realistic. AI is not a panacea, and it also comes with challenges, such as the need for big data, ethical concerns, and the risk of bias in algorithms.
Development of Industry 4.0 Industry 4.0 has continued to evolve since its inception. The vision of fully networked, automated and optimized production is getting closer. Companies have begun to reap the benefits of I4.0 by digitizing their production processes and integrating intelligent systems that increase efficiency and flexibility.
Comparing AI and I4.0 AI and I4.0 are closely linked, as AI is often seen as the driving force behind I4.0. AI enables machines and systems to learn and optimize on their own, which is a core principle of I4.0. Comparing the two shows that while they are different concepts, together they have the potential to revolutionize the industrial landscape.
Should companies invest in AI? The decision to invest in AI should not be taken lightly. Organizations need to consider their goals, resources, and the maturity of their current technologies. AI can bring huge benefits, but it also requires careful planning and implementation. Experts advise adopting a diversified investment strategy that invests in both AI companies and AI technologies to spread risk and benefit from the development of the entire sector.
AI and I4.0 are undoubtedly transformative technologies that have the potential to transform industry. However, the current hype should be viewed critically, and companies should make an informed decision when it comes to investing in AI. It's a time of great opportunity, but also a time when careful consideration and strategic planning are essential.
The ethical concerns in the development and application of artificial intelligence (AI) are diverse and complex. Here are some of the main concerns:
Data protection and privacy: AI systems often require large amounts of data to function effectively. This raises questions about how this data is collected, used, and protected, especially when it comes to personal or sensitive information.
Accountability: When AI systems make decisions that have a negative impact, it is often difficult to determine who is responsible – the developer, the operator, or the AI itself.
Transparency: Many AI systems are designed as "black boxes," which means that their decision-making processes are not transparent. This can lead to suspicion and makes it difficult to check the systems for fairness and accuracy.
Discrimination and bias: AI systems can mirror or even reinforce existing human biases when trained with biased data. This can lead to unfair results, especially in sensitive areas such as hiring staff or lending.
Social impact: Automation through AI can lead to significant changes in the labor market, including job losses. There are also concerns about the impact of AI on social interactions and human relationships.
Security: AI systems can be hacked or tampered with, leading to security risks. There are also concerns about the use of AI in military applications and autonomous weapons.
These concerns are why organizations like UNESCO have created guidelines and recommendations for the ethical development and use of AI. These aim to ensure that AI is used for the benefit of society and that human rights are respected.
Yes, there are numerous examples of commercially successful AI applications that use different business models. Here are some examples and how their business models work:
• AI in content marketing: AI technologies such as ChatGPT are used to generate content and assist in content creation. These models use AI to personalize customer outreach and optimize marketing campaigns.
• AI in customer care: Chatbots and automated customer service tools use AI to answer customer queries and provide support. This results in more efficient customer support and cost savings for the company.
• AI in manufacturing: AI is used to detect anomalies in production and optimize processes. This improves the quality of the products and reduces downtime and costs.
• AI in search engines: Search engines use AI algorithms to deliver relevant search results and improve the user experience. This increases user engagement and generates advertising revenue.
• Smart home with AI: AI is used in smart home devices such as voice assistants to personalize the user experience and offer new services.
These business models are based on automating processes, increasing efficiency, personalizing customer experiences, and improving competitiveness. Companies that successfully use AI define clear goals, collect and analyze the right data, select appropriate AI technologies, and implement them step by step.
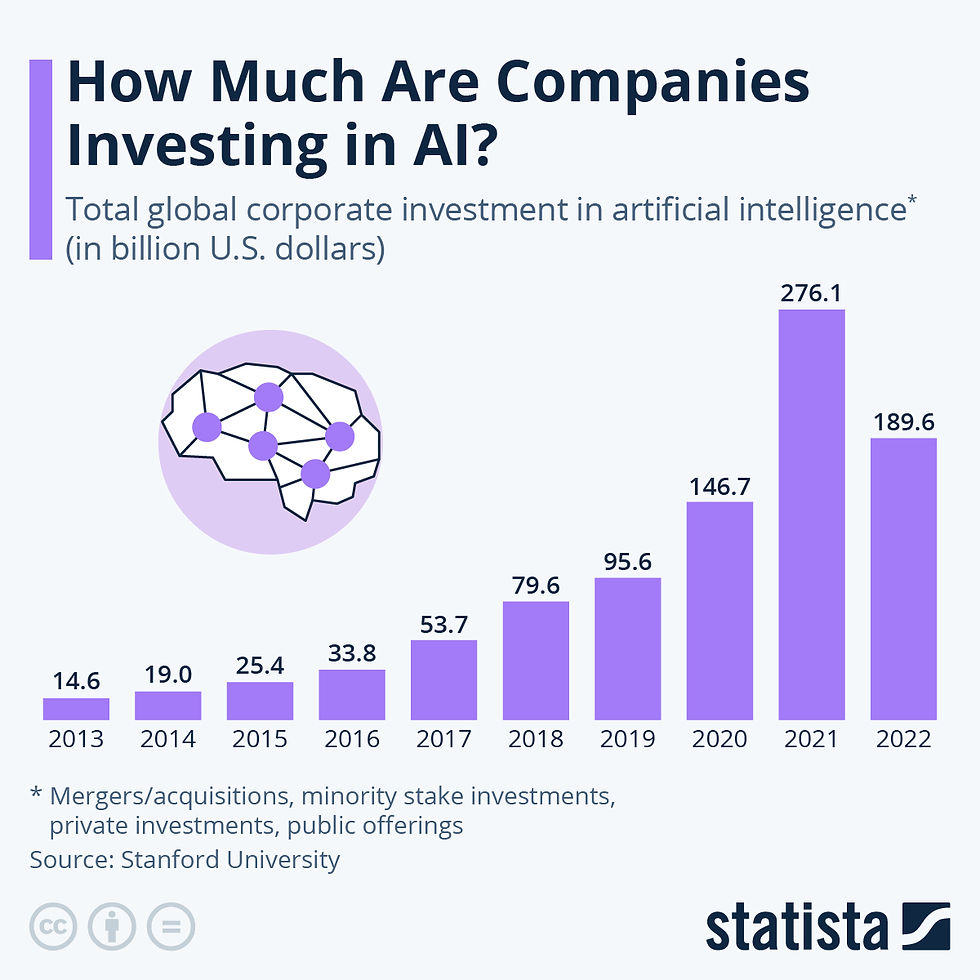
However, there are also examples of AI projects that have not achieved the success they had hoped for. Some of the reasons AI projects fail include:
Superfluous: Many AI projects do not offer any real added value over existing solutions or are not innovative enough to prevail in the market.
Market concentration: Big tech companies are developing their own AI tools and integrating them into their products, making it difficult for smaller vendors to compete.
Lack of buyers: Some AI projects don't find enthusiastic buyers because they either compete with established vendors or offer limited value.
Technical challenges: AI projects can fail due to technical difficulties, such as integrating with existing systems or scaling the technology.
Lack of data: Without sufficient and high-quality data, AI systems cannot be trained effectively, resulting in inaccurate or unusable results.
Ethics and regulation: Ethical concerns and regulatory hurdles can also contribute to the failure of AI projects.
An example of a platform that collects failed AI projects is the "AI Graveyard", which contains a list of projects that have not been successful for various reasons. These examples show that it is important to learn from mistakes and to carefully plan and implement AI projects.
Companies can use various strategies to minimize risks when introducing artificial intelligence (AI):
1. Clear objective: Companies should have a clear idea of what they want to achieve with AI. This includes defining measurable goals and determining how success will be evaluated.
2. Understanding of AI: It is important that everyone involved has a basic understanding of AI and its potentials and limitations. Training courses and workshops can help with this.
3. Ensure data quality: Good data is the foundation of successful AI projects. Companies should ensure that they have high-quality and relevant data.
4. Ethics and compliance: Companies must consider ethical guidelines and compliance requirements to ensure that their AI applications are used responsibly.
5. Risk management: Robust risk management that is specifically geared towards AI can help identify and manage potential risks at an early stage.
6. Pilots: Before AI is adopted on a large scale, pilots can provide valuable insights into how the technology works and how it will impact.
7. Interdisciplinary teams: Involving experts from different fields can help to get a more comprehensive picture of the impact of AI and avoid blind spots.
8. Partnerships: Working with experienced partners and service providers can help to benefit from their knowledge and experience and develop solutions together.
9. Scalability and integration: Companies should make sure that AI solutions are scalable and can be seamlessly integrated into existing systems and processes.
10. Monitoring and evaluation: Continuous monitoring and regular evaluations are necessary to monitor the performance of the AI systems and make adjustments if necessary.
By paying attention to these points, companies can take advantage of the opportunities offered by AI while controlling and minimizing risks.
Comments